The Science and Strategy of Segmentation
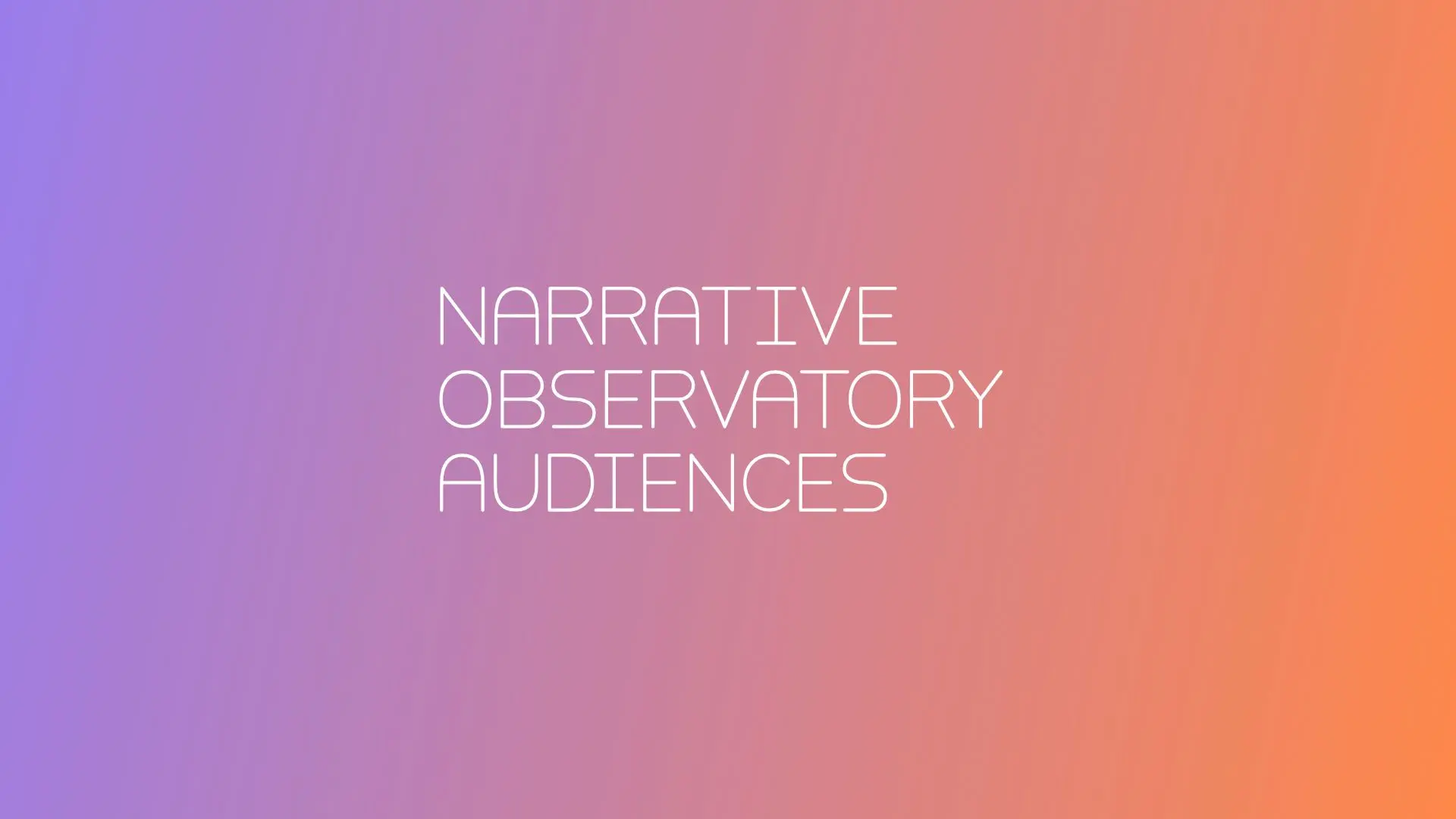
2024-02-07
AI is not a new technology. Stories about “thinking machines” have been around for hundreds of years and even the more contemporary idea of artificial intelligence dates back to 1955, when emeritus Stanford Professor John McCarthy described it as “the science and engineering of making intelligent machines.” Today, it permeates our societal structures and shapes how we engage with media—think Google’s search engines, Siri and Alexa voice assistants, Netflix and Amazon recommendation algorithms, and social media feeds. At a basic level, these traditional forms of AI are problem solving tools programmed to intake and structure big datasets in order to output something useful, like predictions or recommendations.
Generative AI takes artificial intelligence capabilities further. While traditional AI is about classifying and aggregating data that already exists, generative AI is about amalgamating data to produce content that has characteristics resembling the original inputs, most often in the form of text, images, music, audio, and video. And now, the wide availability of generative AI tools—many of which are open source—is enabling people and organizations worldwide to use AI to create content, code, and other media at unprecedented speed and scale.
Yet, development is outpacing policy—surfacing inevitable blindspots. Despite global efforts to assemble protocols, experts like the OECD’s AI Policy Observatory, Center for AI Safety, and RIL’s Responsible AI Framework are spotlighting the need for AI makers and users to safeguard against risks associated with generative AI use. Among concerns are increased incidents of copyright infringement, more deceptive deepfakes, journalistic errors and misinformation, and an amplification of data bias which could lead to problematic stereotyping. As people increasingly use generative AI within creative work, ethical controversies around AI-authored media and art are emerging.
But it’s not all doom and gloom. With responsible use, like any tool humans create, generative AI can benefit society. We’ve already seen humans use it to streamline software engineering, spark creative ideation, inspire invention—and even save lives. Right now, the global community has the chance to join in a commitment towards AI use and design that minimizes harm and bolsters human potential.
At Harmony, our operational imperative is to serve the public good. To this end, our core values guide everything we do, including choosing and using tools like AI.
Transparency is one of those values. As an organization steeped in data science, Harmony has been using AI in applications from machine learning to natural language processing for many years. With the rise in generative AI, we see now as a moment to both assess our past use and discern how best to choose and use tools in the future.
One thing that remains unchanged is our intention. We believe AI should be used to complement—not replace—human strengths. Like other major organizations such as Comscore, OECD, and Google, we’re committed to centering humans in considering how and when to use AI.
These are the ways we’ve been using AI thus far:
Dimension reduction: We analyze media—thousands, sometimes millions, of pieces of media, to capture, for example, patterns in what reaches and resonates with audiences. The sheer volume and pace of media creation and consumption has become impossible to manually process. So we use large language models (LLMs) in natural language processing to filter, cluster, and characterize media transcripts and other kinds of text, which we then use to suggest distinctive narratives or content ecosystems, for example. Doing this usually also involves human annotators to help us build supervised relevance models for media. These annotators interact with the actual media stories to tell us what narratives they perceive. Often variations in how our annotators perceive and interpret stories can tell us something important about differences and similarities in an audience’s narrative landscape.
Visual summaries: We use AI to help us transform distinctive attributes of media into representative visualizations, which we then review, refine, and incorporate into our tools. This process uses humans, for instance, to analyze the outputs of generative AI descriptions of real media artifacts to identify common themes and keywords. These are then distilled into categories, like setting, tone, and texture, that are used to build prompts, for example, for Midjourney to generate visualizations.
Operational efficiency: Much of our work relies on complex software engineering processes and qualitative analysis. We’ve integrated AI tools into our workflows in order to boost productivity. For example, we’ve used tools like Github Copilot and chatGPT to streamline code. Chatbot search engines have helped create media descriptions. And we’ve used AI to customize the processing of clickstream data. The process of coding with an AI is very similar to the traditional pair-programming model, where two developers share one screen, and write and discuss code together. The near-instant feedback loop between the developer and the AI allows us to review and edit each suggestion in real-time.
Moving forward we are considering the following AI applications:
Amplifying impact: We are exploring how advances in LLMs and generative AI can help our research insights to work harder. We are particularly interested in how AI can help us prototype tools to support early stage content testing and strategy validation, and open new ways to surface media attributes and content patterns.
Building a knowledge repository: We’re learning more all the time about what audiences like, what stories resonate with them, and where they encounter those stories. A conventional report, even with really great design, can only convey so much. We’re excited to explore, for example, creating chatbot tour guides for everything we’ve learned with our partners, so that people can ask about audiences, stories, and media in their own words and learn what we know that can be useful to them.
As the media landscape and the content it carries explode in volume and variety, it will be vital to find new ways to support storytellers in reaching audiences, and digesting data into insights will be an important part of this. Fundamentally, we see AI as one way in.
We are encouraged by its potential to enhance our understanding of media and people’s relationship to it. With cautious optimism, we will continue to explore how AI in all forms can help scale our research, spark our creativity, and boost our efficiency.
Simultaneously, we acknowledge our work is only as good as the data and tools we use. We’re committed to remaining cognizant of AI vulnerabilities and mitigating risks wherever possible.
In a follow-up post we will explore in more detail how our core values will shape our continued engagement with AI. As we develop our policies and principles for AI at Harmony Labs, we welcome your input. Please get in touch with any questions or ideas.
Acknowledgment: When writing this piece we consulted with the Harmony Labs Advisory Board and drew inspiration from a plethora of coalitions, think tanks, and industry peers, including the OECD, Responsible Innovation Lab, YouTube, Google, Meltwater, Comscore, and Wired, in addition to US and EU initiatives.