A New Thanksgiving Recipe
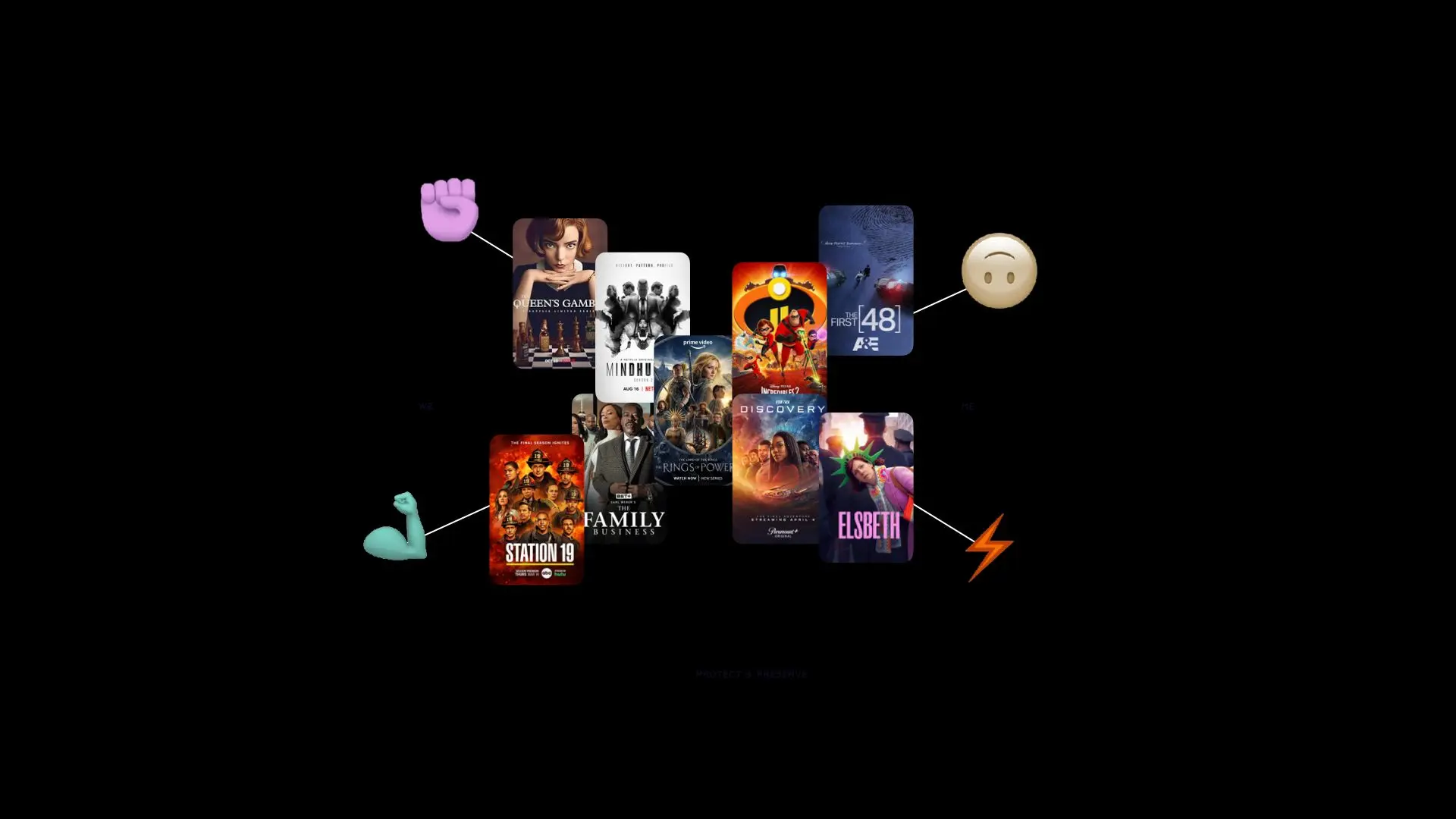
2024-07-22
At Harmony Labs, we view acquiring and studying precise audience data as a prerequisite to understanding culture and affecting change within it. This approach is grounded in the simple truth that just because media content exists, doesn’t mean people are engaging with it. That’s why, instead of taking a more top-down approach that scans a swath of news headlines and social media for some keyword, we channel our resources bottom-up—first identifying and then analyzing all of the content that people are actually reading, viewing, and listening to.
To this end, we’ve put a tremendous amount of thought and work into not only getting the right data, but maximizing its utility. Our data infrastructure isn’t a simple story of acquisition; it's also about the complex engineering processes and pipelines that clean, validate, and enhance that data, especially as today’s media landscape continues to grow, along with the need to filter out noise, like the onslaught of bot activity.
Since our last post on our data infrastructure in 2021, we’ve continued to build a core data set with pretty incredible breadth, diversity, and scale. Combining news, social media, film, and TV—we have vantage into the full text and imagery of content that reflects real audience consumption patterns. This enables our partners, through us, to pursue research queries both macro and micro in nature. Not only can we peer into the broad strokes of media ecosystems, we can also measure user-level interactions—examining prompts like: What storyscapes support inclusive societies? How do people in perceived media deserts get civic information? How can climate communications become more culturally relevant?
We acquire data from a variety of sources. Our audience panel data comes from data philanthropy agreements with Nielsen and Comscore. Then we get content data from APIs as well as data from partners like Peakmetrics and TV Eyes. New data and metadata acquisition efforts are also extending our reach into video- and audio-first podcasts and other media. Collectively, these sources are a unique media data asset—giving us a really wide view and letting us look in all the corners of culture.
There’s no doubt that being able to see the media consumption of hundreds of thousands of individuals in the U.S. is powerful, and yet—the real magic for us is the behind the scenes work of strategically engineering ways to connect our data and amplify their potential.
As it exists today, our data resources give us several routes into actionable media insights and audience analytics—e.g. demand side narratives, cross-platform tracking, and media sequence clustering. In laymans’ terms, we can see things like what stories are getting the most traction within specific groups, what audiences are doing across platforms and devices and what their user journeys via search engines look like.
But it didn’t start that way. While having access to large volumes and diverse sources of data is the first step, there’s a lot of engineering that goes into making data usable. As we acquire new data or work to improve our current stock, we anonymize, clean, augment, and systemize them in order to focus on media behaviors of actual humans and filter out noise created by errors and by non-humans, like bots, and other inauthentic actors.
Within the Narrative Observatory, home to our data infrastructure, we build connections between our “consumption” data, that tracks how people move through media, and our “content” data, which shows what stories, topics, themes, and messages retain attention. Take, for instance, news data. They come from news sites, news aggregation sites, and affiliate sites, which collectively make it hard to know if an article that shows up in one place, like nytimes.com, is the same one that shows up on MSN. Our goal is to characterize and track the specific content that audiences are consuming and that means matching specific article text across urls and sources. Just this corner of our overall pipeline took us over a year to build, and now, monthly, we match almost a million news encounters by more than 30,000 panelists to the full text of the articles they read on 705 different domains.
The process of choosing how to connect data is anything but straightforward. There are countless use cases to predict and problems of both scale and performance to solve. Things like figuring out how to account for different timeframes and timezones and which URLs to match require endless decisions that are unglamorous and mission-critical. This level of complexity is also why our platform works best within a consultancy model, rather than self-serve.
When done right, the complexity of creating connected data pays off—giving us a fuller picture of what media behavior really looks like so we can produce reports like our Main Character Energy brief in which we used combined data from social media, streaming TV, and survey to gain insights into what content fuels identity and cultural relevance among Latino audiences. More profound audience and story insights, in turn, mean more actionable opportunities and intervention strategies for media and creative practitioners to work with across a range of critical issues—from climate change to health equity. You can explore more audience research like this in the work gallery on our website.
When dealing with large data pipelines, errors are inevitable. To overcome these, our team works to test, assess, and monitor data batches to look for consistency and gaps. Yet, as the volume of inputs increases, manually keeping a grip on quality becomes more logistically challenging.
This is where our team's advances in automation come in. Through close cross collaboration between our data scientists and engineers, we create expectations for what data should look like. Then we develop bespoke quality assurance processes that critically screen and strengthen small batches of data which can be scaled up to safeguard across broader historic and incoming data sets. In doing so, we not only support our own data credibility and workflow efficiency, but also provide feedback loops to our data philanthropy partners that have resulted in improvements in their own products and pipelines.
As part of our mission to produce work for the public good, we respect and prioritize the protection of the data entrusted to us. From our business partners to the individuals who supply that data on the user side, we take a comprehensive and rigorous approach to privacy by anonymizing and encrypting any data containing personally identifiable information. We only ingest, use, and store data that aligns with our work imperatives, and maintain strong security protocols based on the principle of least privilege that limits access to data necessary for their specific tasks.
To minimize the risk of unintentional data breaches or misuse, we participate in intensive, annual independent security audits to challenge and validate our own assumptions and systems, and ensure adherence to best practices in data protection among our team of in-house engineers and data scientists.
In step with these internal efforts, we strive to be transparent about our data in the work we share publicly. This means communicating clearly about any biases, assumptions, and limitations in our sources and methodology.
As we continue to build out and improve our data and take on challenging research provocations with our partners, we are constantly reminded that our work is only as good as our team and our data. We’ve learned that good data, meaning data that truly reflects culture, is so much more than an acquisition game. That’s why in recent years we’ve cultivated more opportunities for closer collaboration between our data science and engineering teams to co-create an infrastructure that is increasingly interconnected and reliable.
It’s through these strides that we’re able to deliver richer, sharper, and more insightful answers to timely and complex briefs. And every time we do so, we get to build infrastructure and tools for longevity—that keep growing alongside our evolving media landscape, well after a project wraps. We’re also committed to making our work public so that the insights this infrastructure allows reach as many people as possible and have maximal impact. Subscribe to our newsletter for future updates about the evolution of our data and tools, and all of our latest research.